11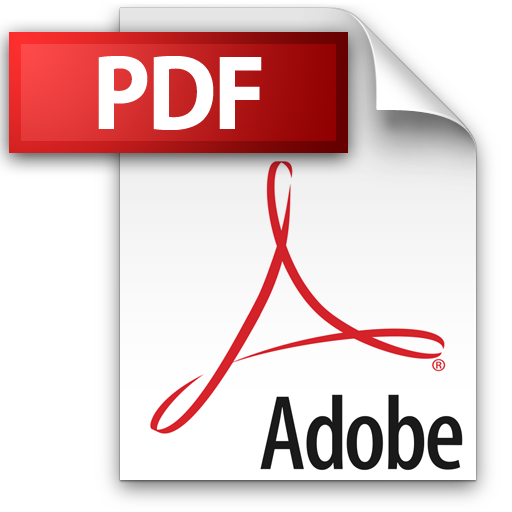 | Add to Reading ListSource URL: users.ics.aalto.fiLanguage: English - Date: 2015-08-21 10:06:56
|
---|
12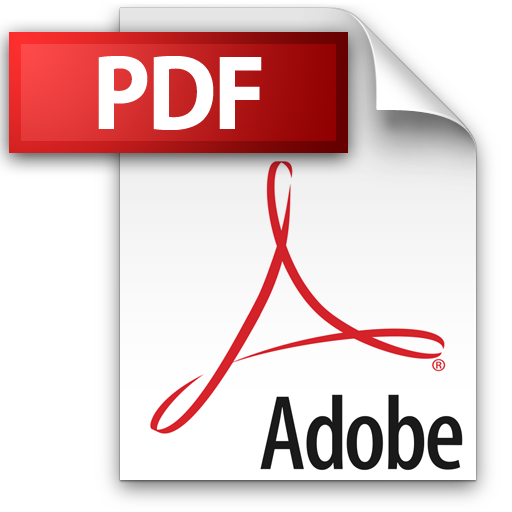 | Add to Reading ListSource URL: www.work.caltech.eduLanguage: English - Date: 2015-01-01 11:20:46
|
---|
13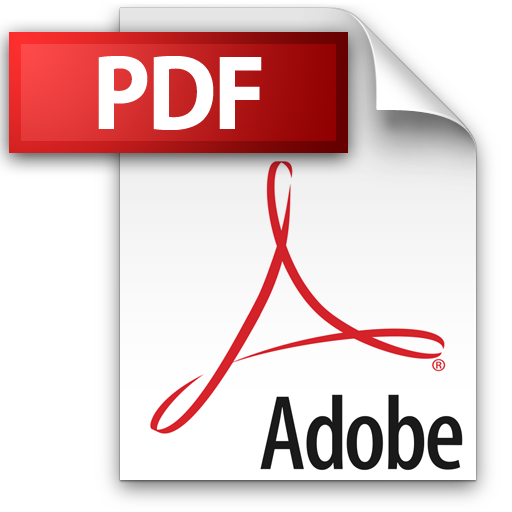 | Add to Reading ListSource URL: jmlr.orgLanguage: English - Date: 2016-07-20 01:41:10
|
---|
14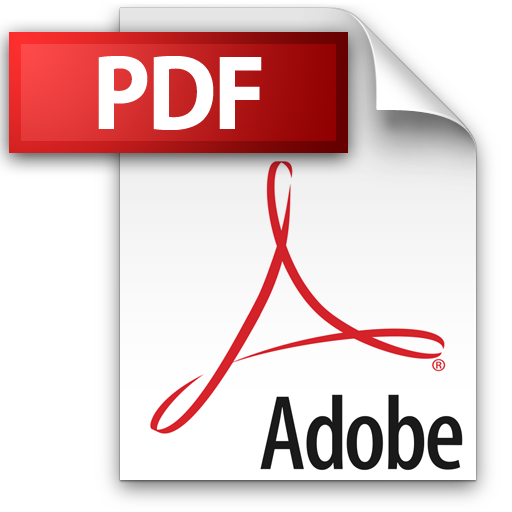 | Add to Reading ListSource URL: jmlr.orgLanguage: English - Date: 2015-07-20 20:08:35
|
---|
15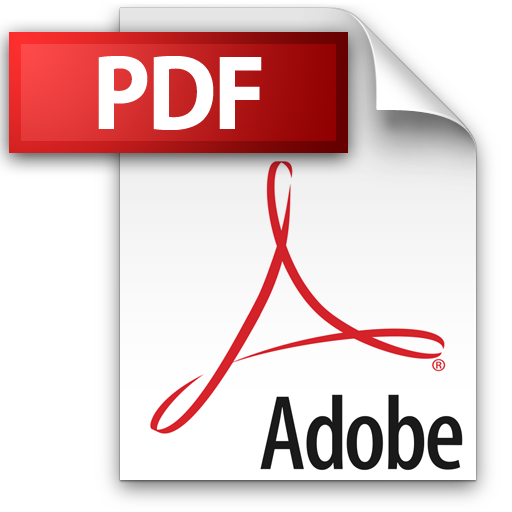 | Add to Reading ListSource URL: www.jmlr.orgLanguage: English - Date: 2011-07-05 16:26:18
|
---|
16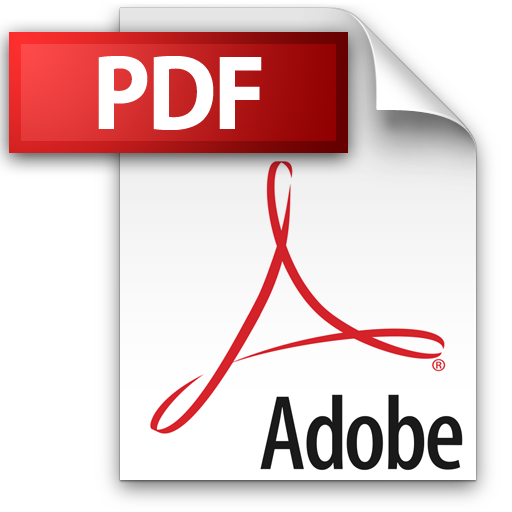 | Add to Reading ListSource URL: arxiv.orgLanguage: English - Date: 2014-02-18 01:51:44
|
---|
17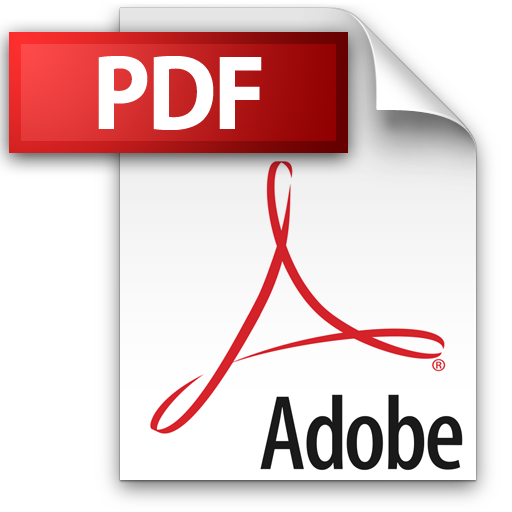 | Add to Reading ListSource URL: users.ics.aalto.fiLanguage: English - Date: 2012-10-25 03:54:39
|
---|
18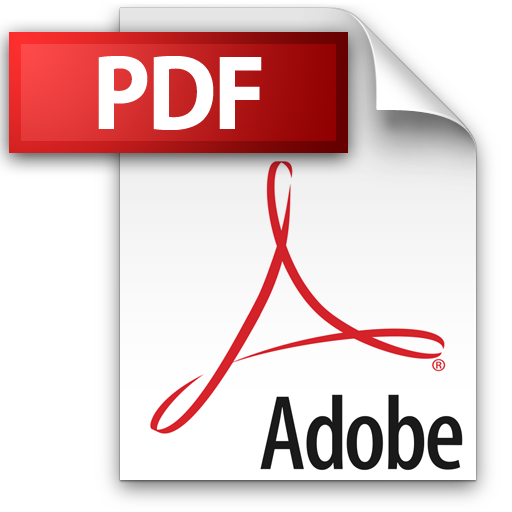 | Add to Reading ListSource URL: theory.stanford.eduLanguage: English - Date: 2016-06-04 09:49:44
|
---|
19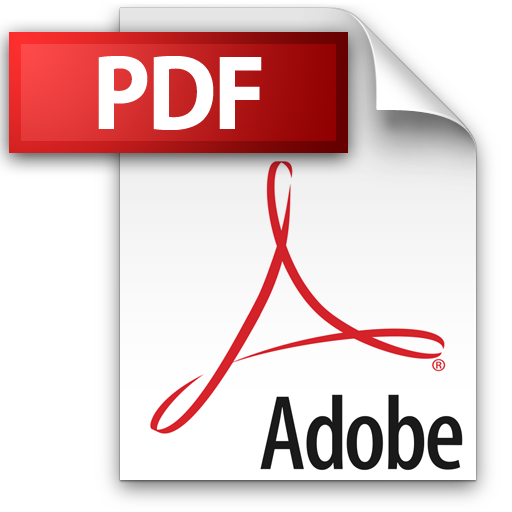 | Add to Reading ListSource URL: jmlr.orgLanguage: English - Date: 2015-07-20 20:08:36
|
---|
20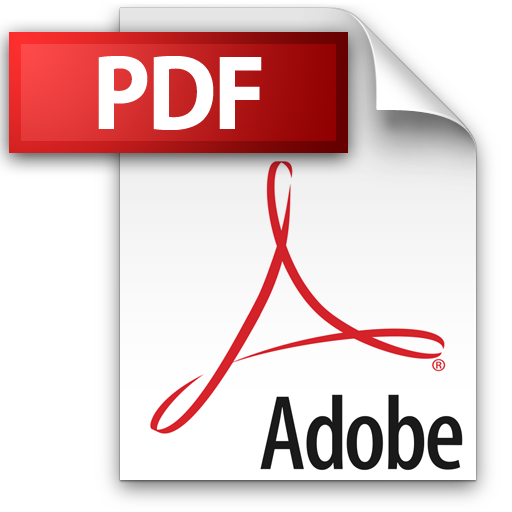 | Add to Reading ListSource URL: www.proba.jussieu.frLanguage: English |
---|